Transforming Healthcare through Medical Data Annotation
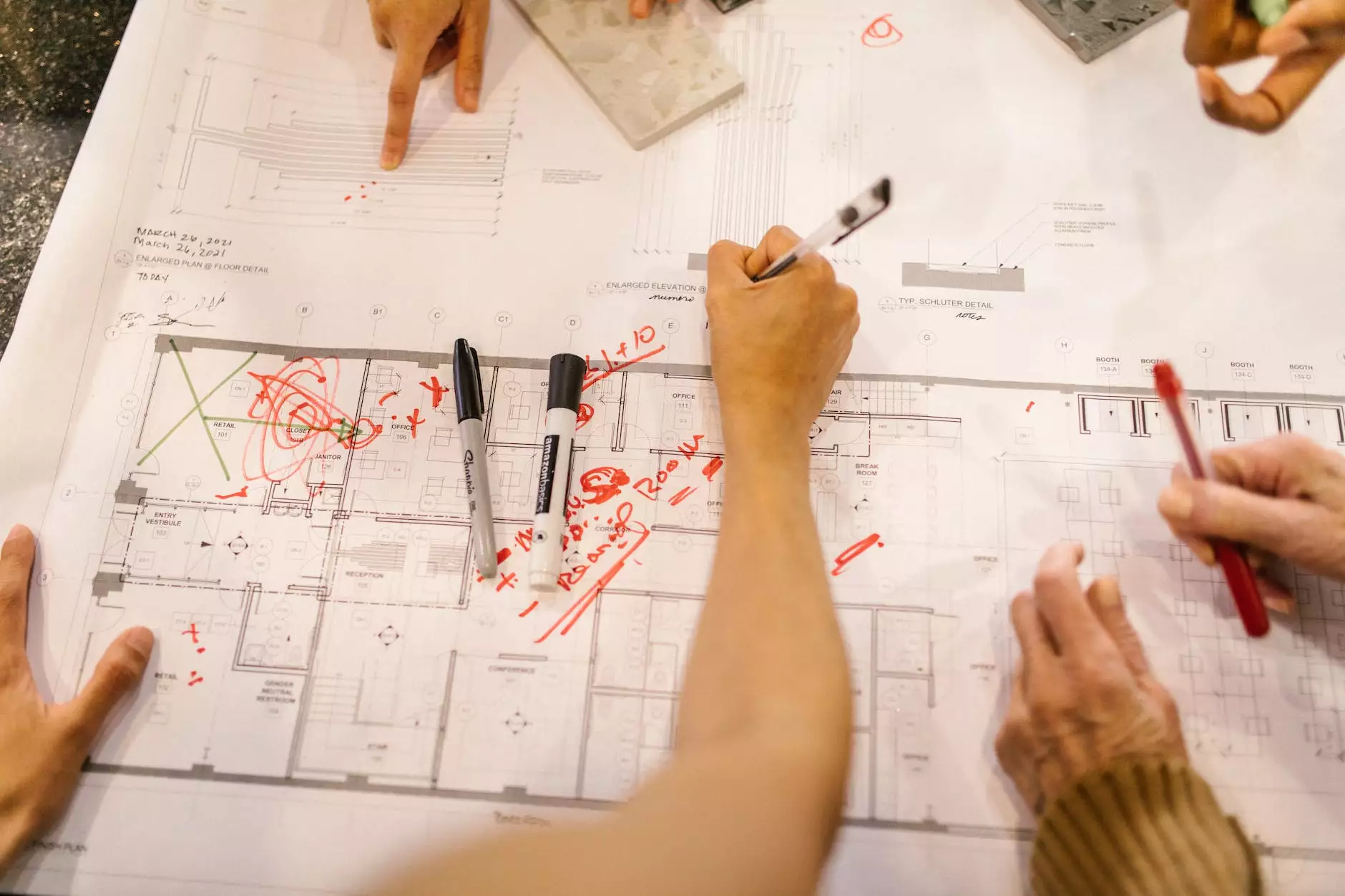
Introduction to Medical Data Annotation
In the rapidly evolving landscape of healthcare, accurate data management is paramount. Medical data annotation serves as the backbone of numerous healthcare technologies, enabling improved decision-making and patient care. This process involves the meticulous tagging and categorization of medical documents, images, and other data types to facilitate better machine learning models and data analysis.
What is Medical Data Annotation?
At its core, medical data annotation is the process of labeling and categorizing medical data to make it comprehensible for algorithms and machine learning models. This involves a variety of data types, including:
- Images: Annotating medical images such as X-rays, MRIs, and CT scans to assist in diagnostics.
- Clinical Records: Tagging patient data in clinical records to identify relevant information easily.
- Biomedical Literature: Indexing research articles and clinical studies for easier reference.
The Importance of Medical Data Annotation in Healthcare
The significance of medical data annotation cannot be overstated. Here are a few reasons why it plays a crucial role in the healthcare industry:
1. Enhancing Diagnostic Accuracy
Annotated medical data allows healthcare providers to utilize advanced AI algorithms that enhance diagnostic accuracy. For instance, in radiology, annotated images can be used to train algorithms to distinguish between healthy and pathological tissues, leading to timely and accurate diagnosis.
2. Supporting Clinical Decision-Making
Reliable annotations enable healthcare professionals to access relevant information rapidly, assisting in clinical decision-making processes. This is particularly vital in emergency situations where swift decisions can impact patient survival.
3. Facilitating Research and Development
Annotated data is indispensable for research and pharmaceutical development. It can be utilized to identify trends, investigate drug interactions, and discover new treatment modalities based on real-world evidence.
Types of Medical Data Annotation
Various methods of medical data annotation can be applied, depending on the data type and intended use. Here are some prevalent types:
1. Image Annotation
This method involves labeling medical images to support diagnostic processes. Common techniques include:
- Bounding Boxes: Drawing boxes around specific areas in an image to indicate a region of interest.
- Segmentation: Dividing an image into segments to facilitate detailed analysis of structures.
- Point Annotations: Marking specific points, such as tumors, to facilitate precise measurements.
2. Text Annotation
Text annotation involves tagging relevant segments of clinical texts, such as discharge summaries and electronic health records. Techniques include:
- Named Entity Recognition: Identifying and classifying entities from text, like symptoms, diagnoses, and medications.
- Sentiment Analysis: Analyzing the sentiment conveyed in patient feedback or notes.
3. Video Annotation
With the increasing use of video in telemedicine and patient monitoring, video annotation has gained traction. It involves labeling frames within videos for:
- Action Recognition: Identifying specific clinical actions taken during patient interaction.
- Behavior Analysis: Analyzing patient behavior for better treatment approaches.
Challenges in Medical Data Annotation
Despite its critical role, medical data annotation comes with several challenges:
1. Data Privacy and Security
Handling sensitive medical data necessitates strict adherence to privacy laws and regulations, such as HIPAA in the United States. Ensuring data anonymity while annotating poses significant challenges.
2. High Volume of Data
The sheer volume of medical data generated daily can be overwhelming. Efficiently managing this data for annotation purposes requires innovative technological solutions.
3. Quality Assurance
The effectiveness of machine learning models depends heavily on the quality of annotated data. Ensuring that annotations are accurate and consistent is a challenge that needs continual addressing.
The Future of Medical Data Annotation
The future of medical data annotation shines brightly as technology continues to advance. Here are some trends shaping the future of this field:
1. Integration of AI and Automation
Automation tools powered by artificial intelligence are being developed to streamline the annotation process. These tools can significantly reduce the time required for annotation and enhance accuracy.
2. Crowdsourced Annotation
Crowdsourcing annotation tasks can provide a wider array of insights and perspectives. This is particularly useful in areas like genomics and personalized medicine, where diverse data sources can enhance research outcomes.
3. Emphasis on Real-World Evidence
There is a growing emphasis on utilizing real-world evidence to inform clinical practices and drug development. Annotating real-world data will become increasingly critical for understanding patient outcomes and treatment efficacy.
Conclusion
Medical data annotation is a transformative force in the healthcare sector, proving pivotal for diagnostics, clinical decision-making, and research. As we navigate forward, the importance of precise, reliable, and comprehensive data annotation will only continue to amplify. Businesses seeking to enhance patient care and streamline operations must invest in robust data annotation practices and technologies. This approach will not only improve patient outcomes but also position organizations at the forefront of healthcare innovation.
Why Choose KeyMakr for Your Medical Data Annotation Needs?
At KeyMakr.com, we understand the unique challenges posed by medical data annotation. Our team leverages cutting-edge technology and expert knowledge to deliver accurate and efficient annotation services tailored to your specific needs. With a commitment to quality and compliance with industry regulations, we ensure that your data management processes enhance patient care while maintaining the highest standards of privacy and security.