Unlocking the Power of Image Labeling for Object Detection
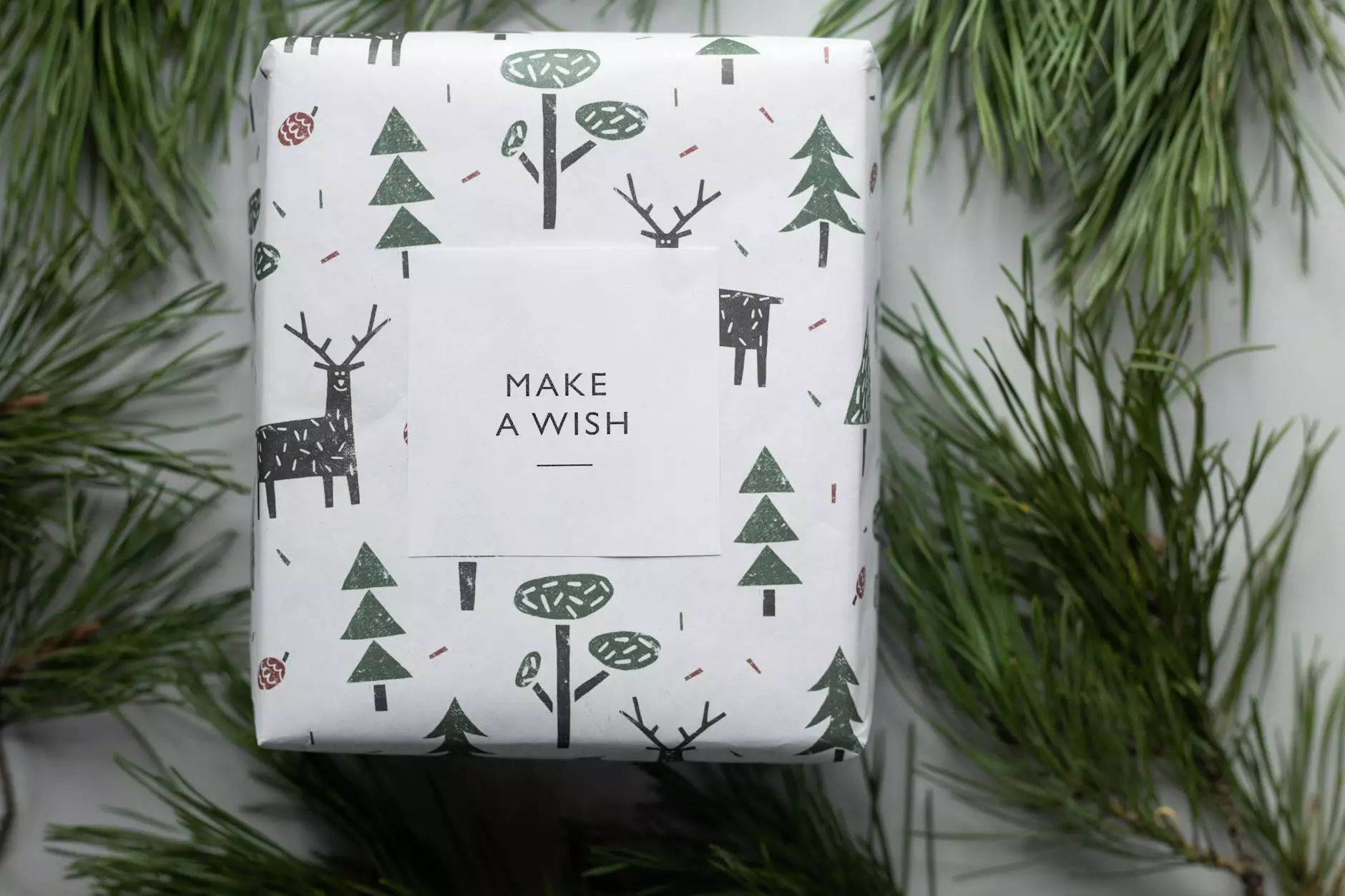
In today's rapidly advancing technological landscape, businesses are increasingly reliant on artificial intelligence systems capable of recognizing and classifying objects within images. The effectiveness of these systems is heavily dependent on one crucial process: labeling images for object detection. In this article, we will delve deep into this essential methodology, explore the tools available, and understand how a robust data annotation platform can elevate your business's AI capabilities.
Understanding Object Detection
Object detection is not just a buzzword; it is a vital process in comprehending and utilizing visual data. This aspect of computer vision allows software to see and identify objects in images or video feeds. This process can be broken down into a few fundamental steps:
- Input Data Collection: Gathering images that represent real-world scenarios.
- Data Labeling: Annotating images to highlight different objects.
- Model Training: Using annotated data to train AI models.
- Prediction: Applying trained models to new images for object recognition.
The Importance of Labeling Images for Object Detection
Labeling images is the cornerstone of any object detection project. A high-quality dataset with accurate labels directly influences the performance of AI models. Here’s why it is critical:
1. Accuracy of Models
Without precise labels, the AI models cant learn effectively. Models trained on poorly labeled datasets suffer from low accuracy, resulting in poor performance in real-world applications.
2. Diversity in Data
A diverse dataset encapsulates various conditions under which the objects might appear. Factors such as lighting, angles, and backgrounds significantly affect object detection. Therefore, labeling various scenarios helps robust model training.
3. Enhancing AI Learning
When images are properly labeled for object detection, AI can learn to differentiate between similar objects, enhancing its capability to interpret complex scenes. This would lead to advanced functionalities in applications such as autonomous vehicles, security monitoring, and more.
Key Labs AI: A Leader in Data Annotation
When it comes to reliable and efficient data annotation, Key Labs AI stands out with its robust data annotation tools and platforms. Designed for ease of use and equipped with advanced functionalities, Key Labs AI helps businesses swiftly scale their AI projects.
Features of Key Labs AI's Data Annotation Tool
- User-Friendly Interface: The annotation tool is designed for simplicity, making it accessible for annotators of all skill levels.
- Customizable Labeling Options: Users can create custom label sets tailored to specific project requirements.
- Batch Processing: Annotate multiple images simultaneously, significantly reducing project timelines.
- Quality Assurance Mechanisms: Built-in review systems ensure high accuracy and consistency in labels.
- Integration Capabilities: Easily integrate with existing machine-learning frameworks and pipelines.
How to Effectively Label Images for Object Detection
Getting the most out of your labeling process requires planning and structure. Here’s a structured approach to doing it right:
1. Define Clear Objectives
Before starting, identify the goals of your object detection model. Understanding what you want to achieve will guide you in gathering the right images and selecting appropriate labels.
2. Develop a Labeling Guideline
Create a detailed guideline that outlines how objects should be labeled. This should consider factors like:
- Object boundaries
- Overlapping objects
- Label formats
- Quality standards
3. Choose the Right Annotation Type
There are different types of annotations such as bounding boxes, polygons, and segmentation masks. The choice depends on the complexity of the objects in your images.
4. Utilize Advanced Annotation Tools
Adopting a high-end data annotation tool like Key Labs AI can greatly simplify the labeling process. This ensures efficiency and accuracy while minimizing the potential for human errors.
5. Conduct Regular Reviews and Quality Checks
Once the images are labeled, it’s crucial to implement a review process. Employing annotation teams and utilizing quality checks will help maintain the integrity of your dataset.
Real-World Applications of Object Detection
The applications of object detection are vast, spanning across various industries. Here are some notable examples:
1. Automotive Industry
Self-driving cars leverage object detection to identify pedestrians, other vehicles, traffic signs, and obstacles, enhancing safety and driving efficiency.
2. Healthcare
In medical imaging, object detection plays a critical role in recognizing anomalies such as tumors in X-rays and MRIs, facilitating quicker diagnoses and treatment planning.
3. Retail
Businesses use object detection for inventory management, security surveillance, and improving customer experience by analyzing shopping behaviors.
4. Agriculture
Farmers utilize object detection technologies for monitoring crops' health, identifying weeds, and automating harvesting processes.
Challenges in Labeling Images for Object Detection
While labeling images is essential, it comes with its unique set of challenges, including:
1. Time Consumption
Manual labeling of images is often labor-intensive and time-consuming, particularly for large datasets.
2. Skill Level Variability
The quality of annotations can vary significantly based on the annotator's skill level, which can lead to inconsistencies within the dataset.
3. Complexity of Scenarios
Images with numerous overlapping or similar objects pose additional difficulties, making accurate labeling challenging.
Future of Image Annotation and Object Detection
The future of image annotation and object detection is promising, with advancements in machine learning and AI leading the way. Here are some trends to watch:
1. Automation in Annotation
Increasing reliance on AI to automate parts of the labeling process will enhance efficiency and reduce human error.
2. Enhanced Tools and Platforms
Continued improvements in annotation tools will make the process more efficient, providing features like real-time collaboration and cloud integration.
3. Growing Demand Across Industries
As technology evolves, the demand for precise object detection will rise across various sectors, propelling industries to invest in better annotation practices and tools.
Conclusion
In conclusion, labeling images for object detection serves as a vital foundation for developing sophisticated AI systems capable of performing complex tasks across numerous industries. Key Labs AI’s advanced data annotation tools and platforms provide businesses an unprecedented opportunity to excel in their AI endeavors. By investing in high-quality image labeling practices, businesses can unlock the full potential of their AI models, leading to improved efficiency, accuracy, and innovation in their fields.
If you’re ready to enhance your image annotation processes and drive your business towards AI excellence, explore Key Labs AI today and capitalize on the power of high-quality data annotation.