Labeling Training Data: The Comprehensive Guide to Data Annotation Success
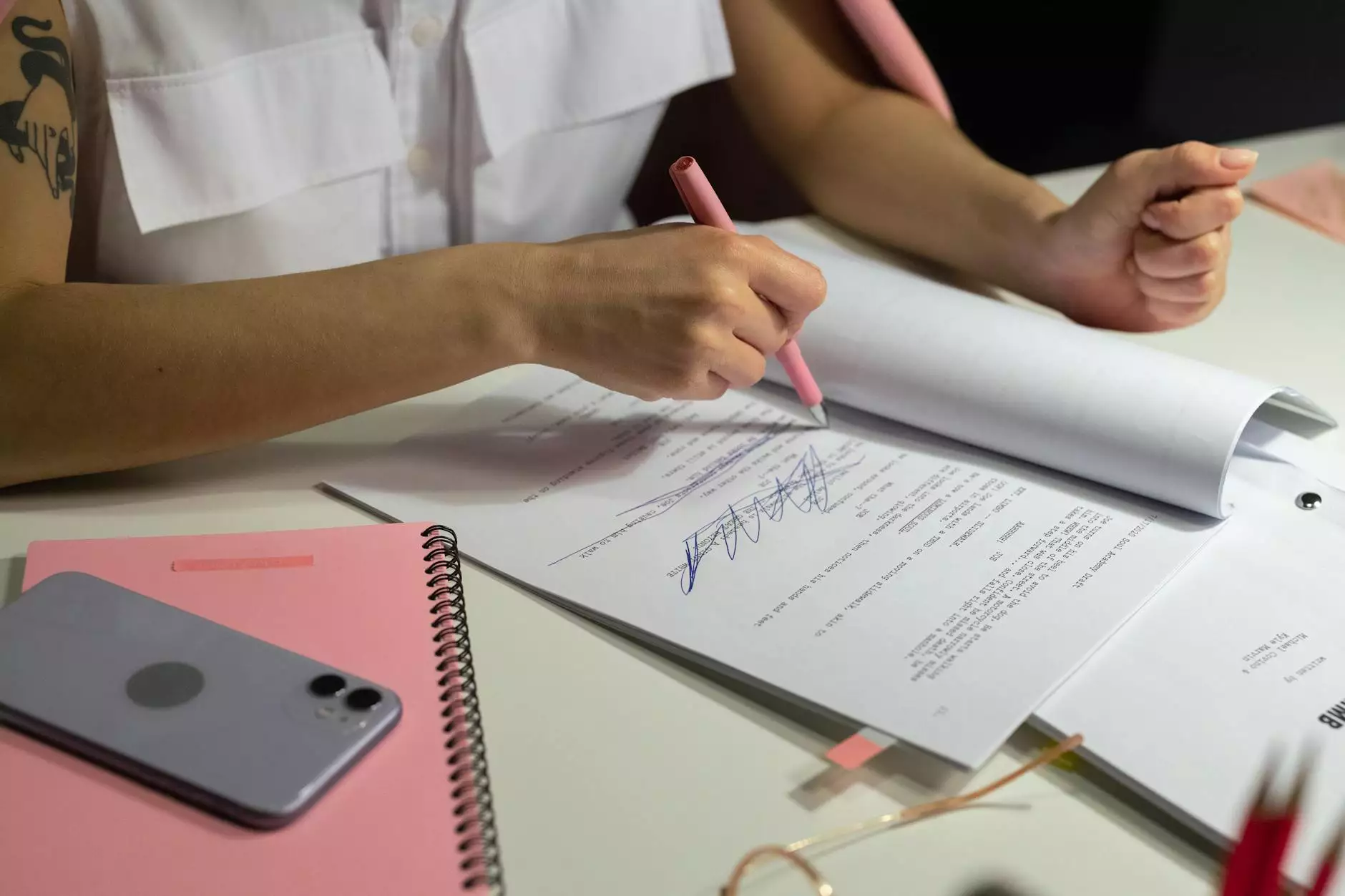
Understanding the Importance of Labeling Training Data
Labeling training data is a fundamental aspect of machine learning and artificial intelligence. It refers to the process of providing clear and concise annotations to datasets so that algorithms can learn from labeled examples. This process is crucial for supervised learning, where the model learns to make predictions or decisions based on labeled training data.
In an era where data is often termed as the "new oil," effective data annotation becomes key in unlocking its potential. The growth of AI-driven technologies has led to an increased demand for high-quality labeled data, effectively making data annotation platforms a linchpin in the development of machine learning models.
What is Data Annotation?
Data annotation is the process of tagging or labeling data with relevant information. This process allows AI algorithms to understand the context of the data and train accordingly. There are various forms of data annotation, including:
- Image Annotation: Tagging images for object detection, segmentation, and recognition.
- Text Annotation: Labeling sections of text for sentiment analysis, named entity recognition, and more.
- Video Annotation: Annotating frames in videos to identify patterns and actions.
- Audio Annotation: Labeling audio clips for speech recognition and emotion detection.
Why is Labeling Training Data Critical?
The quality of your labeled training data directly influences the performance of your machine learning models. Poorly labeled data can lead to inaccurate predictions, while well-annotated data can significantly improve model accuracy and reliability.
Here are a few reasons why labeling training data is critical:
- Accuracy: Models trained on high-quality labeled data tend to perform better.
- Efficiency: Properly labeled data accelerates the training phase, reducing the time taken to reach optimal performance.
- Scalability: Well-structured datasets can be easily expanded or modified to include new data points.
Choosing the Right Data Annotation Tool
As the demand for high-quality labeling training data increases, choosing the right data annotation tool becomes essential. When selecting a data annotation tool, consider the following factors:
1. User Interface and Usability
A user-friendly interface allows annotators to work more efficiently. Tools that are easy to navigate significantly reduce the time spent on annotation tasks.
2. Supported Data Types
Ensure that the annotation tool supports the specific type of data you are working with, whether it's images, text, video, or audio. Some tools specialize in certain formats.
3. Collaboration Features
If you are working in a team, collaboration features such as real-time updates, comments, and task assignments can enhance productivity and communication.
4. Automation Capabilities
Tools that provide automation can significantly reduce the manual effort required for data labeling. Look for features like semi-automated annotation that uses AI to assist human annotators.
5. Quality Assurance
Robust quality assurance processes are crucial in ensuring data integrity. Look for a tool that allows for review and feedback mechanisms, enabling annotators to improve over time.
Keylabs.ai: Your Partner in Data Annotation
At Keylabs.ai, we understand the intricacies of labeling training data. Our data annotation tools and platforms are designed to meet the needs of businesses looking to leverage AI effectively.
Features of Keylabs.ai
- Multi-Format Support: Our platform supports various data types including images, text, videos, and audio, ensuring that you can annotate all your data in one place.
- Advanced Automation: With cutting-edge machine learning models, Keylabs.ai offers semi-automated annotation to enhance productivity without sacrificing quality.
- Collaboration Tools: Our tools allow teams to collaborate seamlessly, enabling efficient task management and feedback loops.
- Quality Assurance Checks: Keylabs.ai incorporates built-in quality checks to ensure the accuracy and reliability of your labeled datasets.
Best Practices for Labeling Training Data
When embarking on your data annotation journey, following best practices can ensure your outcomes are optimal:
1. Define Clear Guidelines
Create comprehensive annotation guidelines that define exactly what each label means. This ensures consistency across annotations and reduces ambiguity.
2. Train Your Annotators
Proper training for annotators is essential. Training helps to ensure they understand the guidelines and can apply them correctly.
3. Monitor Quality
Regularly review annotated data to ensure it meets quality standards. Use feedback to improve the process continuously.
4. Use Diverse Datasets
Diverse data samples ensure that your model learns from a broad spectrum of inputs, improving its robustness and performance in real-world scenarios.
5. Iterate and Improve
Data annotation is an iterative process. Continuously refine your guidelines and tools based on feedback and results from your models.
The Future of Labeling Training Data
The future of labeling training data lies in continued advancements in AI and automation. As technology evolves, we can expect:
1. Enhanced Automation Tools
As AI becomes more sophisticated, automation tools will be able to handle more complex tasks in the data labeling process, reducing the human workload and increasing overall efficiency.
2. Greater Integration with Machine Learning
The integration of data annotation tools with machine learning platforms will allow for a smoother workflow—from data collection to model deployment.
3. Focus on Ethical AI
As the AI industry pushes toward ethical considerations, the importance of well-labeled data that reflects diverse perspectives and avoids bias will become even more paramount.
Conclusion
In conclusion, labeling training data is a pivotal step in developing effective machine learning models. By utilizing advanced data annotation tools like those offered by Keylabs.ai, businesses can ensure that their data is accurately annotated, leading to better AI outcomes.
Investing time and resources into labeling training data is not merely a task—it's a strategic move that can dramatically influence how well your AI performs. By adhering to best practices and choosing the right tools, companies can harness the full potential of their data and stay ahead in this rapidly evolving digital landscape.
For more information on how Keylabs.ai can assist you in your data annotation journey, visit our website today.